Enhancing Language Model Performance: Microsoft's Prompt Wizard Revolution
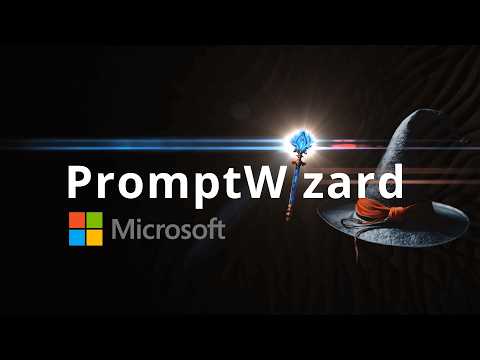
- Authors
- Published on
- Published on
In this riveting video from Sam Witteveen, the focus is on the critical importance of optimizing prompts for language models like LLMs. Viewers are taken on a thrilling ride through the world of context and input quality, showcasing how these factors directly impact the output quality of these models. Enter Microsoft's cutting-edge framework, Prompt Wizard, a game-changer in the realm of prompt optimization. This revolutionary tool automates and simplifies the process, aiming to elevate the performance of language models to unprecedented levels.
Prompt Wizard is not just another run-of-the-mill tool; it's a powerhouse of innovation. By leveraging feedback-driven refinement, joint optimization, and self-generated Chain of Thought steps, this framework pushes the boundaries of what language models can achieve. With a focus on evolving instructions and in-context learning examples over time, Prompt Wizard sets a new standard in prompt engineering. Microsoft's dedication to excellence shines through as they tackle the challenge of prompt optimization head-on, aiming to revolutionize the way we interact with language models.
As the video delves deeper into the inner workings of Prompt Wizard, viewers are treated to a behind-the-scenes look at how this framework operates. From refining prompt instructions to generating diverse synthetic examples, Prompt Wizard leaves no stone unturned in its quest for optimal performance. The framework's iterative approach and emphasis on feedback ensure that prompt optimization is a dynamic and ever-evolving process. With Prompt Wizard at the helm, the future of prompt engineering looks brighter than ever before.
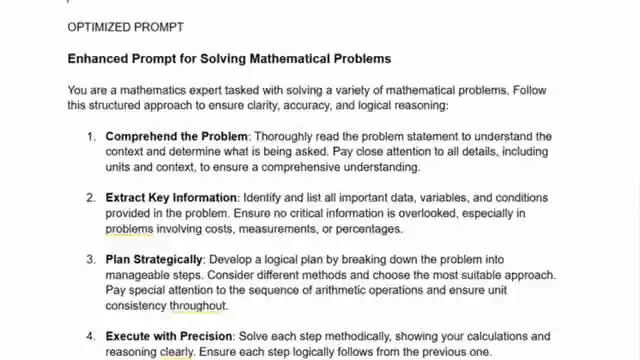
Image copyright Youtube
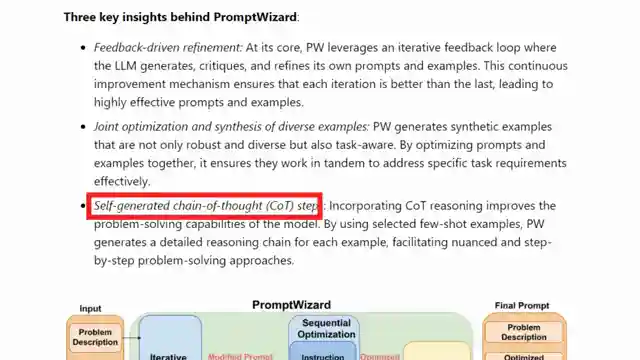
Image copyright Youtube
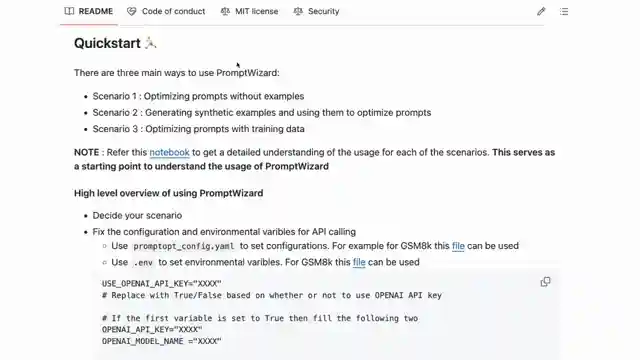
Image copyright Youtube
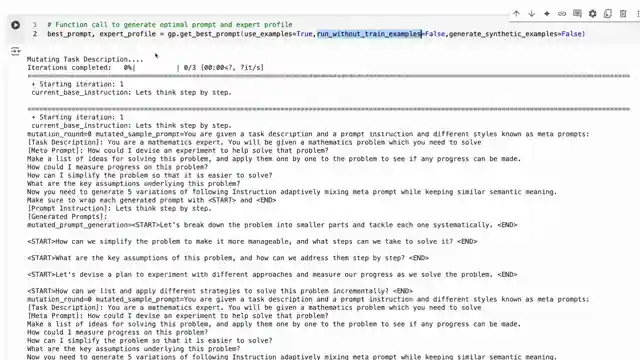
Image copyright Youtube
Watch How to OPTIMIZE your prompts for better Reasoning! on Youtube
Viewer Reactions for How to OPTIMIZE your prompts for better Reasoning!
Comparison between PromptWizard and other tools like textgrad and dspy
Concerns about token usage and cost
Feasibility of developing a similar prompt optimization tool independently
Handling of real-time context variables in prompts
Use of large prompts in production and preference for multiple smaller prompts
Request for examples of human prompt improvement
Cost and token usage of PromptWizard
Effectiveness of PromptWizard compared to fine-tuning a model
Use of genetics algorithm in the iterative optimization process
Difficulty faced by models under 8B with long prompts
Related Articles
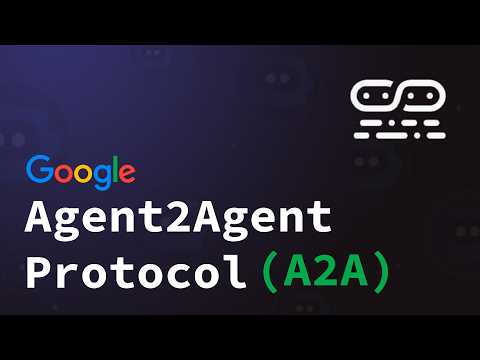
Exploring Google Cloud Next 2025: Unveiling the Agent-to-Agent Protocol
Sam Witteveen explores Google Cloud Next 2025's focus on agents, highlighting the new agent-to-agent protocol for seamless collaboration among digital entities. The blog discusses the protocol's features, potential impact, and the importance of feedback for further development.
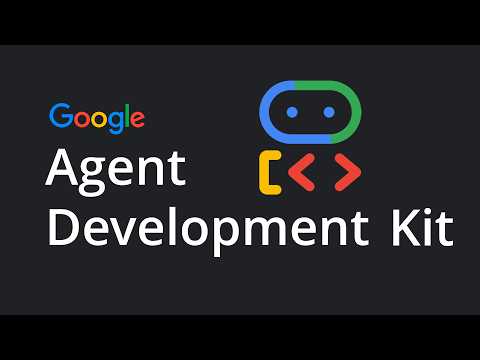
Google Cloud Next Unveils Agent Developer Kit: Python Integration & Model Support
Explore Google's cutting-edge Agent Developer Kit at Google Cloud Next, featuring a multi-agent architecture, Python integration, and support for Gemini and OpenAI models. Stay tuned for in-depth insights from Sam Witteveen on this innovative framework.
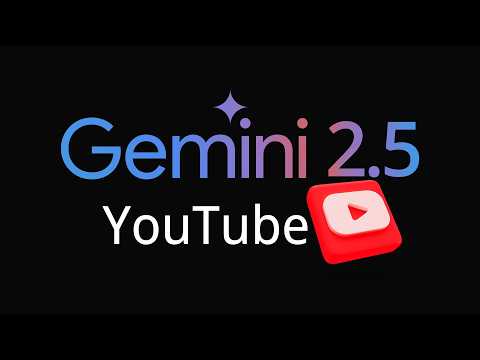
Mastering Audio and Video Transcription: Gemini 2.5 Pro Tips
Explore how the channel demonstrates using Gemini 2.5 Pro for audio transcription and delves into video transcription, focusing on YouTube content. Learn about uploading video files, Google's YouTube URL upload feature, and extracting code visually from videos for efficient content extraction.
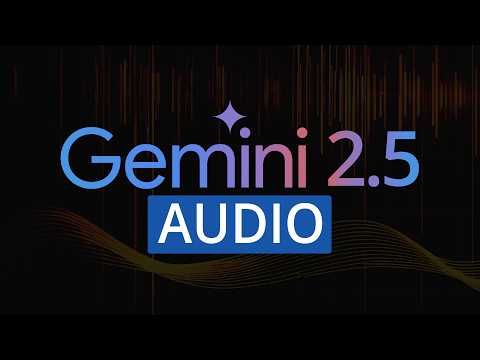
Unlocking Audio Excellence: Gemini 2.5 Transcription and Analysis
Explore the transformative power of Gemini 2.5 for audio tasks like transcription and diarization. Learn how this model generates 64,000 tokens, enabling 2 hours of audio transcripts. Witness the evolution of Gemini models and practical applications in audio analysis.