Deep Seek R1 Model: Unleashing Advanced AI Capabilities
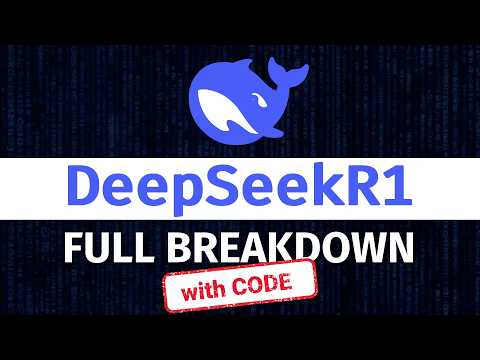
- Authors
- Published on
- Published on
Deep Seek unveiled the R1 light preview model, leaving everyone in awe. This week, they unleashed a whole family of models, including the Deep 60 and distilled models, which outperformed big names like GPT-40. The MIT-licensed Deep Seek R1 model is a game-changer, allowing users to train other models with its outputs. A detailed paper delves into the model's groundbreaking techniques, setting it apart from the competition.
In benchmarks, the Deep Seek R1 model shines, even surpassing the OpenAI 01 model in some instances. Leveraging the Deep Seek V3 base model, the R1 model showcases a unique approach to post-training, yielding exceptional results. The model's performance on the chat.deepseek.com demo app demonstrates its impressive thinking process and reasoning abilities, handling various questions with finesse.
The technical paper reveals the model's evolution, with the Deep Seek R1 benefiting from reinforcement learning training to enhance its capabilities. Through a multi-stage training pipeline, including fine-tuning and reinforcement learning, the model's performance continues to impress. Additionally, distillation techniques have been employed to create smaller models from the Deep Seek R1, showcasing the model's adaptability and versatility in the AI landscape.
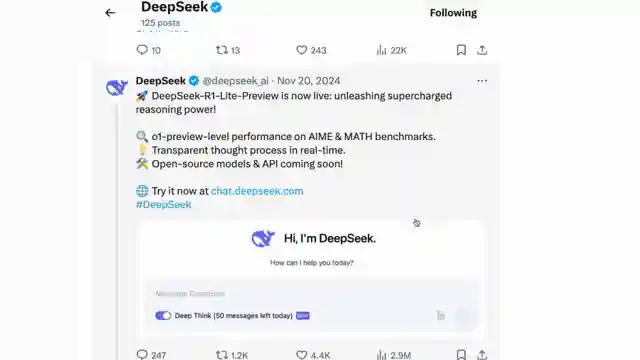
Image copyright Youtube
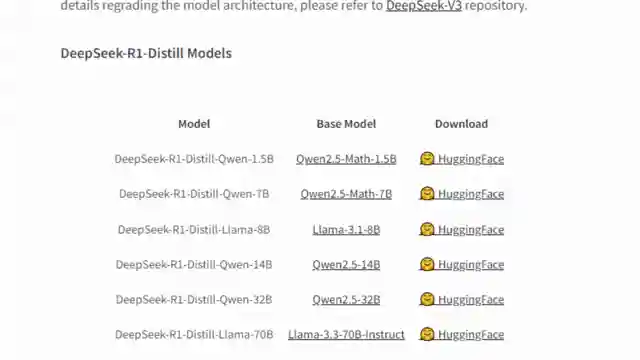
Image copyright Youtube
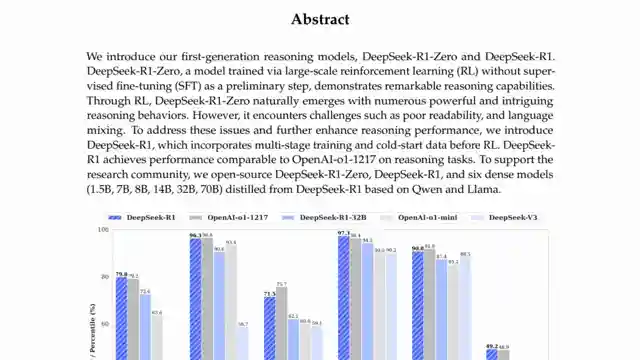
Image copyright Youtube
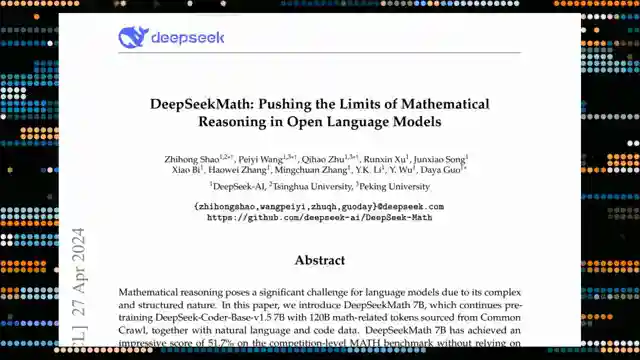
Image copyright Youtube
Watch DeepSeekR1 - Full Breakdown on Youtube
Viewer Reactions for DeepSeekR1 - Full Breakdown
The usefulness and technical details of the DeepSeek R1 model are appreciated
Discussion on Generalized Advantage Estimation (GAE) and its relation to adaptive control systems
Mention of the model's multilingual capability and suggestions for testing reasoning
Comments on the model's performance and capabilities compared to other models
Questions about the model's distillation procedure and running distilled models
Praise for the video content and explanation provided
Concerns and comparisons between open-source and proprietary models
Questions about the use of supervised fine-tuning and reinforcement learning in AI development
Comments on political aspects related to China and the U.S.
Speculation on the impact of OpenAI's methods on other AI companies
Related Articles
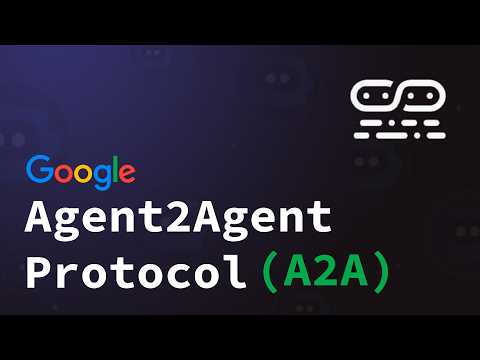
Exploring Google Cloud Next 2025: Unveiling the Agent-to-Agent Protocol
Sam Witteveen explores Google Cloud Next 2025's focus on agents, highlighting the new agent-to-agent protocol for seamless collaboration among digital entities. The blog discusses the protocol's features, potential impact, and the importance of feedback for further development.
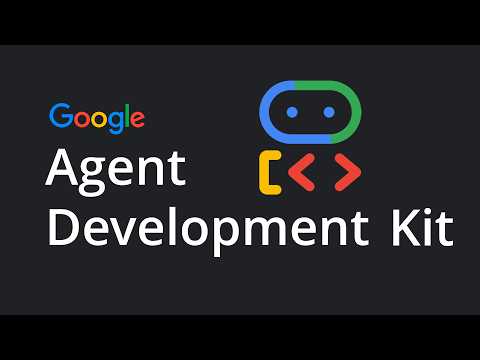
Google Cloud Next Unveils Agent Developer Kit: Python Integration & Model Support
Explore Google's cutting-edge Agent Developer Kit at Google Cloud Next, featuring a multi-agent architecture, Python integration, and support for Gemini and OpenAI models. Stay tuned for in-depth insights from Sam Witteveen on this innovative framework.
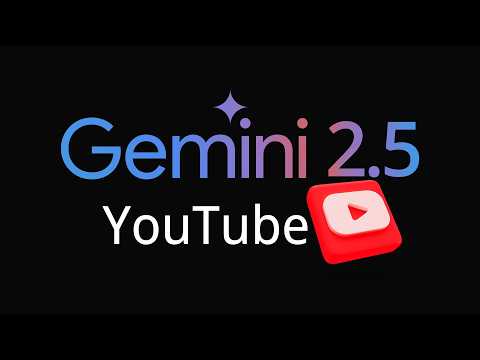
Mastering Audio and Video Transcription: Gemini 2.5 Pro Tips
Explore how the channel demonstrates using Gemini 2.5 Pro for audio transcription and delves into video transcription, focusing on YouTube content. Learn about uploading video files, Google's YouTube URL upload feature, and extracting code visually from videos for efficient content extraction.
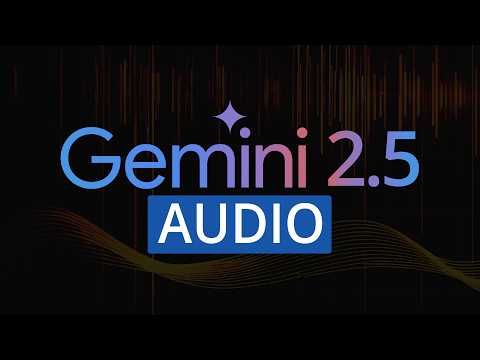
Unlocking Audio Excellence: Gemini 2.5 Transcription and Analysis
Explore the transformative power of Gemini 2.5 for audio tasks like transcription and diarization. Learn how this model generates 64,000 tokens, enabling 2 hours of audio transcripts. Witness the evolution of Gemini models and practical applications in audio analysis.