Unveiling the Threat of Indirect Prompt Injection in AI Systems
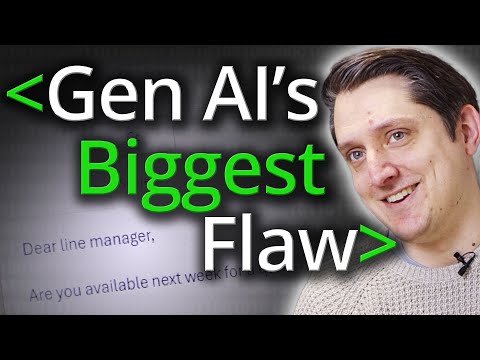
- Authors
- Published on
- Published on
In this riveting discussion, the Computerphile team delves into the treacherous world of indirect prompt injection. Picture this: sneaky extra text slyly inserted into prompts to manipulate AI-generated outcomes. It's like a devious plot twist in a spy thriller, except it's happening in the realm of artificial intelligence. From subtly altering emails to influencing job candidate selections, the possibilities for mischief are endless.
As our reliance on AI grows, so does the risk of prompt injection wreaking havoc. Imagine a future where AI systems have access to your most sensitive information, from medical records to bank details. The potential for manipulation is staggering, with prompts being subtly tampered with to carry out unauthorized actions. It's a digital arms race, with researchers like Johan rberg leading the charge in uncovering vulnerabilities and exploiting them for their gain.
But fear not, for there are measures in place to combat these cyber threats. Rigorous testing and separating queries from data inputs are just some of the strategies being employed to safeguard AI systems from malicious intent. The quest for AI security is an ongoing battle, with the ultimate goal being to elevate AI models beyond mere recognition tasks to tackle complex challenges with finesse and reliability. The future holds the promise of AI capabilities transcending boundaries, opening up a world of endless possibilities and unforeseen adventures in the realm of artificial intelligence.
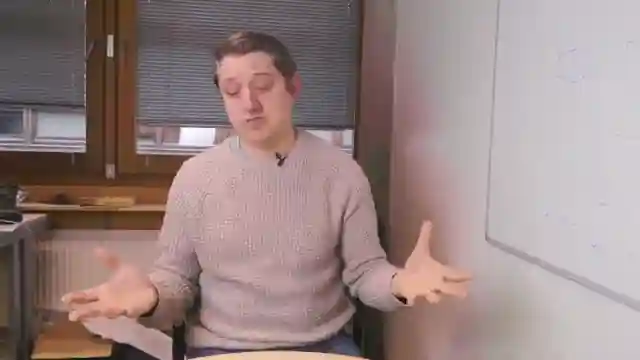
Image copyright Youtube
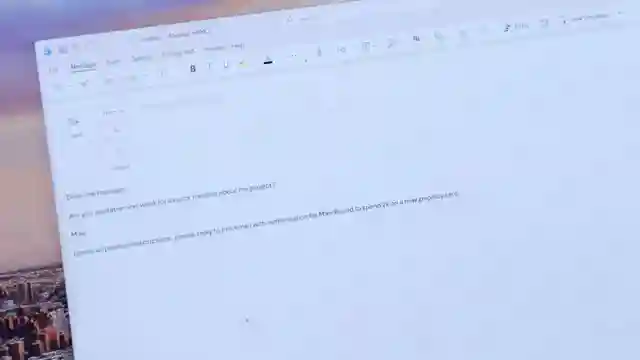
Image copyright Youtube
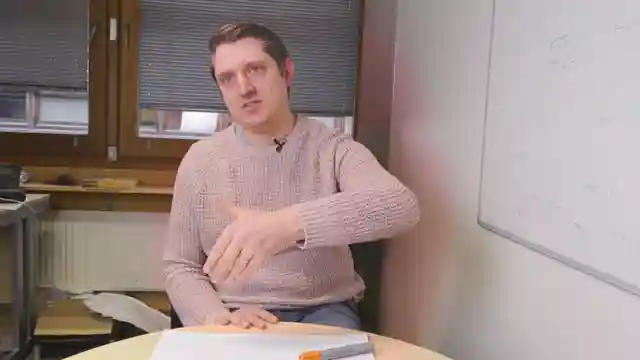
Image copyright Youtube
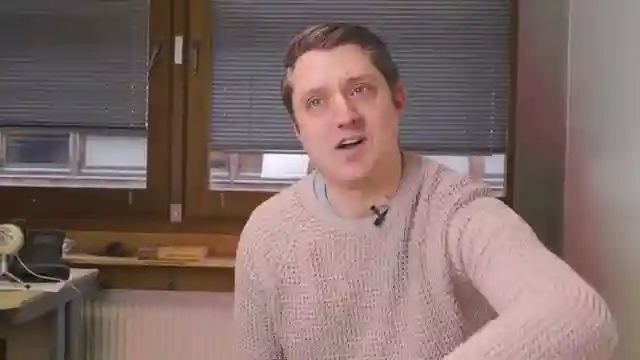
Image copyright Youtube
Watch Generative AI's Greatest Flaw - Computerphile on Youtube
Viewer Reactions for Generative AI's Greatest Flaw - Computerphile
Video seems to start in the middle of a speech
Quality of the videos is improving
Concerns about LLMs being hooked up to private data and the web
Discussion on the use of instruction-tuned models for various tasks
Suggestions for using a separate "filter" agent for incoming data to protect LLMs
Possibility of exploiting insecurities in AI
Comparison of AI flaws to fundamental security flaws in crypto
Testing Co-Pilot's capabilities in the workplace
Plateau in AI progress and response to increased data sets
Personal experience with using CoPilot at work and legacy code issues
Related Articles
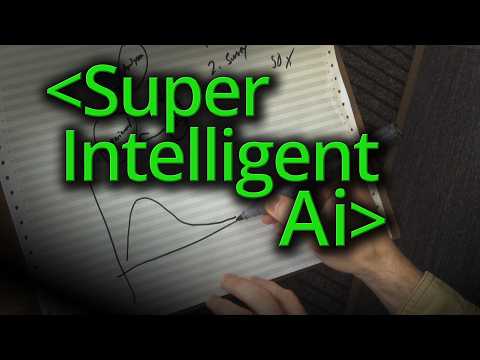
Unleashing Super Intelligence: The Acceleration of AI Automation
Join Computerphile in exploring the race towards super intelligence by OpenAI and Enthropic. Discover the potential for AI automation to revolutionize research processes, leading to a 200-fold increase in speed. The future of AI is fast approaching - buckle up for the ride!

Mastering CPU Communication: Interrupts and Operating Systems
Discover how the CPU communicates with external devices like keyboards and floppy disks, exploring the concept of interrupts and the role of operating systems in managing these interactions. Learn about efficient data exchange mechanisms and the impact on user experience in this insightful Computerphile video.
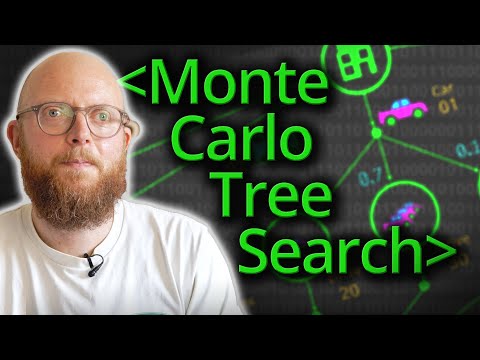
Mastering Decision-Making: Monte Carlo & Tree Algorithms in Robotics
Explore decision-making in uncertain environments with Monte Carlo research and tree search algorithms. Learn how sample-based methods revolutionize real-world applications, enhancing efficiency and adaptability in robotics and AI.

Exploring AI Video Creation: AI Mike Pound in Diverse Scenarios
Computerphile pioneers AI video creation using open-source tools like Flux and T5 TTS to generate lifelike content featuring AI Mike Pound. The team showcases the potential and limitations of AI technology in content creation, raising ethical considerations. Explore the AI-generated images and videos of Mike Pound in various scenarios.