Unlocking Data Efficiency: The Magic of Vector Databases
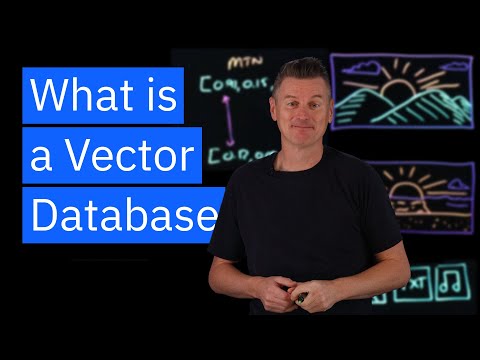
- Authors
- Published on
- Published on
In this riveting episode of IBM Technology, we embark on a thrilling journey into the world of vector databases. Picture this: a digital image of a breathtaking sunset on a mountain vista. But storing this beauty in a traditional relational database falls short in capturing its true essence. Enter vector databases, the unsung heroes representing data as mathematical vector embeddings, allowing for semantic searches and a deeper understanding of unstructured data.
These vector embeddings, arrays of numbers capturing learned features, revolutionize data representation. By training on massive datasets, embedding models extract abstract features layer by layer, unveiling the hidden complexities of images, text, and audio. This process paves the way for powerful operations like similarity searches, made efficient through vector indexing using cutting-edge algorithms like HNSW and IVF.
The heart of vector databases lies in their ability to store and retrieve unstructured data quickly and semantically, shaping the future of information retrieval and generation. Through the RAG feature, vector databases become the cornerstone of retrieval augmented generation, fueling a seamless flow of data for various applications. So buckle up and join us on this exhilarating ride through the realm of vector databases, where data transcends boundaries and possibilities are endless.
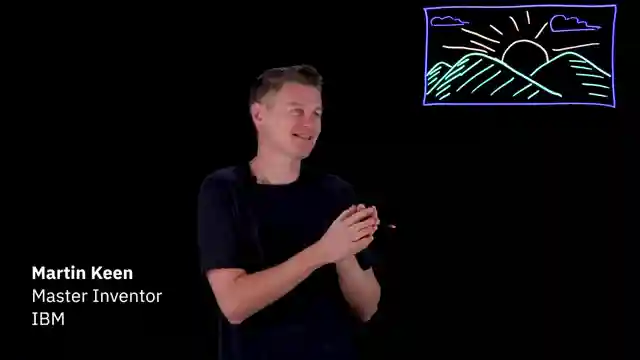
Image copyright Youtube
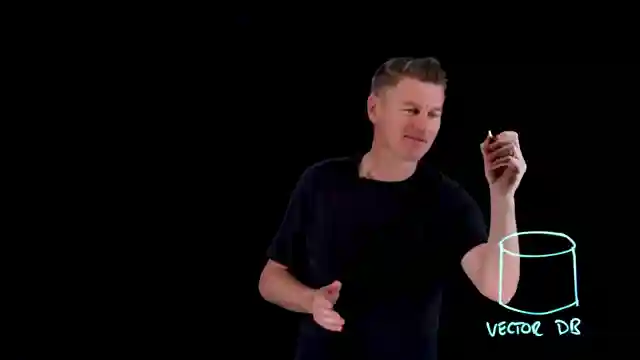
Image copyright Youtube
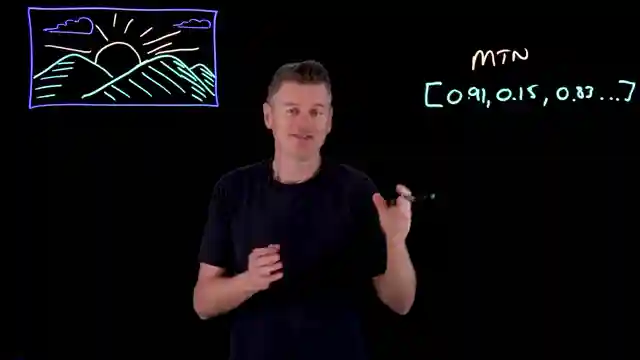
Image copyright Youtube
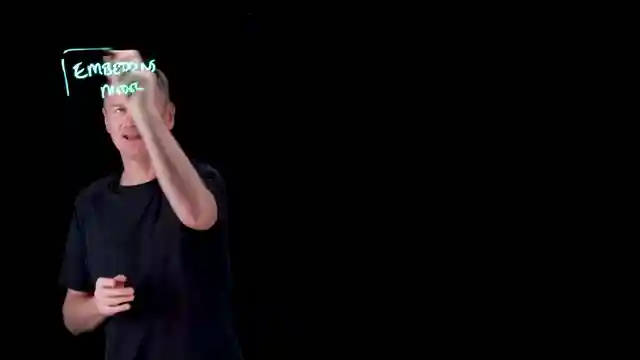
Image copyright Youtube
Watch What is a Vector Database? Powering Semantic Search & AI Applications on Youtube
Viewer Reactions for What is a Vector Database? Powering Semantic Search & AI Applications
Positive feedback on the clear and informative explanation of embeddings and vector databases
Appreciation for the well-done and free videos by IBM
Question about how to 'vectorise' audio/sound
Concern about the future of SQL with the rise of semantic queries
Related Articles
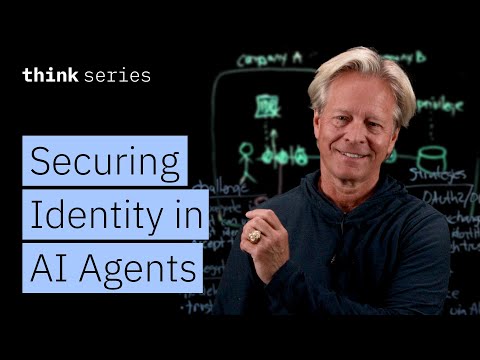
Mastering Identity Propagation in Agentic Systems: Strategies and Challenges
IBM Technology explores challenges in identity propagation within agentic systems. They discuss delegation patterns and strategies like OAuth 2, token exchange, and API gateways for secure data management.
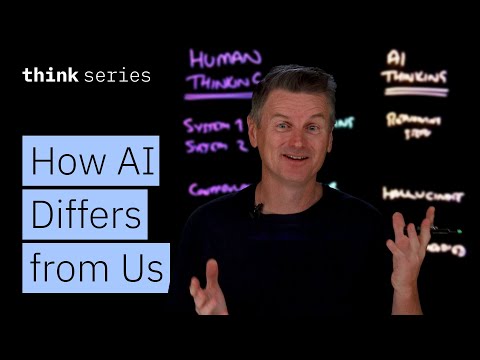
AI vs. Human Thinking: Cognition Comparison by IBM Technology
IBM Technology explores the differences between artificial intelligence and human thinking in learning, processing, memory, reasoning, error tendencies, and embodiment. The comparison highlights unique approaches and challenges in cognition.
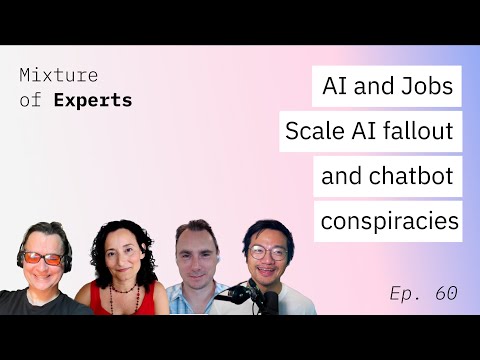
AI Job Impact Debate & Market Response: IBM Tech Analysis
Discover the debate on AI's impact on jobs in the latest IBM Technology episode. Experts discuss the potential for job transformation and the importance of AI literacy. The team also analyzes the market response to the Scale AI-Meta deal, prompting tech giants to rethink data strategies.
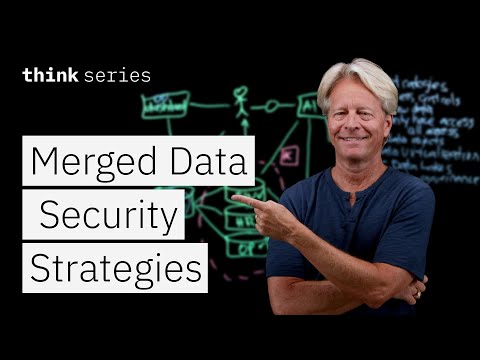
Enhancing Data Security in Enterprises: Strategies for Protecting Merged Data
IBM Technology explores data utilization in enterprises, focusing on business intelligence and AI. Strategies like data virtualization and birthright access are discussed to protect merged data, ensuring secure and efficient data access environments.