Revolutionizing Data Extraction: Alama's Structured Outputs and Vision Models
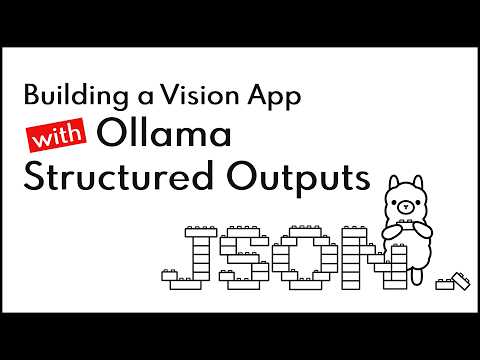
- Authors
- Published on
- Published on
In this riveting episode from the channel Sam Witteveen, the team delves into the thrilling world of structured outputs in Alama. This groundbreaking addition allows for a structured passing of text and data extraction from images, revolutionizing the way tasks are handled. With the introduction of structured outputs, Python users can now set up classes with pantic to finely tune how outputs are structured, providing a level of control like never before. The team showcases code examples, illustrating how this feature can be utilized for simple tasks and even building apps using a vision model to extract valuable information. This is the kind of innovation that gets the adrenaline pumping, offering a glimpse into the future of AI technology.
The video emphasizes the beauty of simplicity, highlighting the fact that complex agent frameworks are not always necessary. By directly writing Python or JavaScript code, users can tailor their applications to perform specific tasks efficiently. Moreover, the ability to leverage large language models locally without relying on external APIs opens up a world of possibilities. The demonstration of extracting entities using classes and validating structured outputs showcases the power and precision of this new feature. It's like witnessing a high-speed race where every move is calculated and executed flawlessly.
Furthermore, the comparison between different versions of Alama models sheds light on the iterative process of fine-tuning for optimal results. The team's exploration of analyzing images of bookshelves and extracting book details using custom prompts and the Alama 3.2 Vision model adds a thrilling dimension to the discussion. The potential of extracting track listings from album covers without the need for an agent framework is a testament to the versatility and ingenuity of this technology. By structuring outputs with descriptions and nesting objects, the team demonstrates how to extract valuable information efficiently. This is the kind of cutting-edge technology that leaves you on the edge of your seat, eager to see what's next in the world of AI.
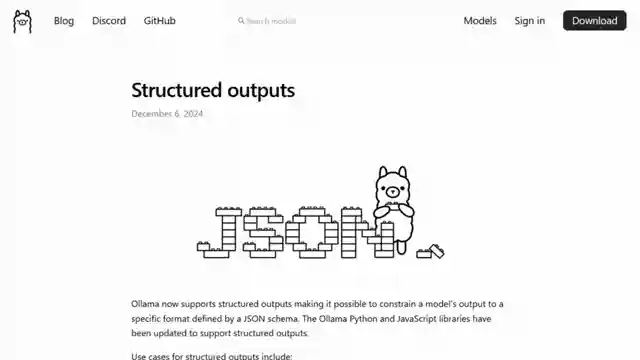
Image copyright Youtube
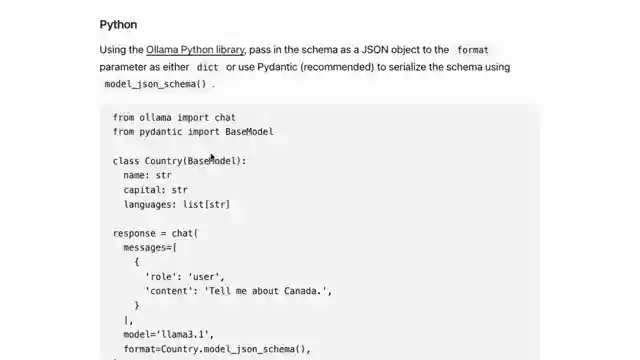
Image copyright Youtube
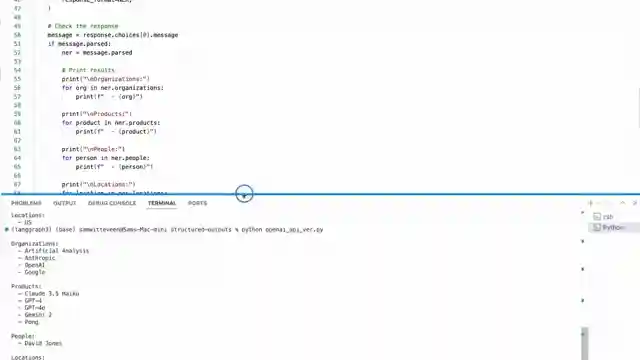
Image copyright Youtube
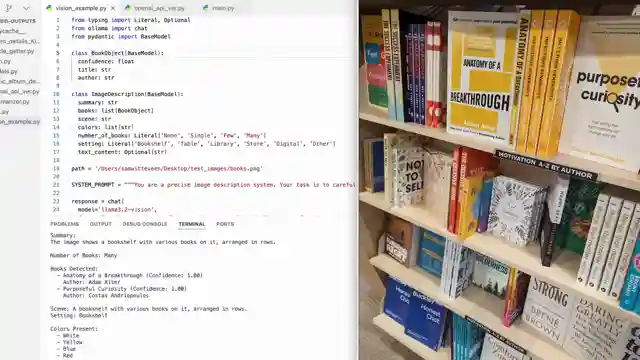
Image copyright Youtube
Watch Building a Vision App with Ollama Structured Outputs on Youtube
Viewer Reactions for Building a Vision App with Ollama Structured Outputs
User learning AI opensource with Python and Ollama
Request for a simple example of fine-tuning a vision model with Ollama
Appreciation for the channel's practical development how-to content
Difficulty in keeping up with new releases
Interest in using NER with LLMs and comparison to SpaCy
Curiosity about using Miles and IA
Request for video on required specs for using LLMs locally
Limitation of Llama vision model to only pictures and structured output
Interest in extracting information from invoices and saving into Excel using structured output
Request for in-depth tutorial on finetuning models for improved accuracy
Question on the possibility of intelligent document processing and classification with open source vision models
Inquiry about using the model for getting coordinates of objects in images
Request for a video on using vision-based models for reading and describing images in a document
Curiosity about the system prompt response and the significance of 2025
Experience with model performance depending on the model itself
Comment on the hacking required for results not being production quality
Request for Hindi audio track availability
Appreciation for the useful content
Request for support of regular expressions with pydantic pattern field
Related Articles
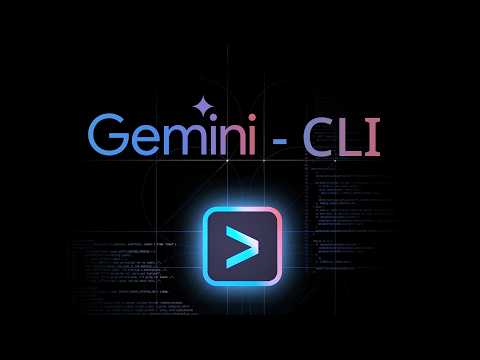
Unleashing Gemini CLI: Google's Free AI Coding Tool
Discover the Gemini CLI by Google and the Gemini team. This free tool offers 60 requests per minute and 1,000 requests per day, empowering users with AI-assisted coding capabilities. Explore its features, from grounding prompts in Google Search to using various MCPS for seamless project management.
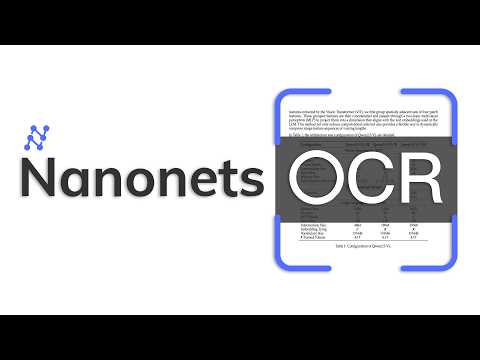
Nanet's OCR Small: Advanced Features for Specialized Document Processing
Nanet's OCR Small, based on Quen 2.5VL, offers advanced features like equation recognition, signature detection, and table extraction. This model excels in specialized OCR tasks, showcasing superior performance and versatility in document processing.
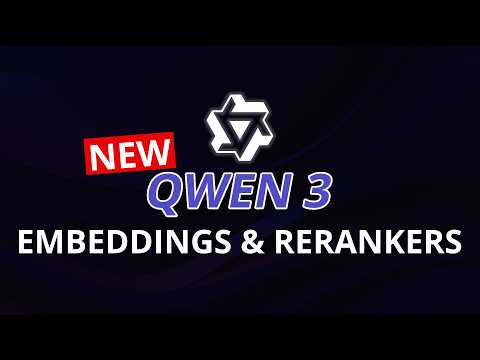
Revolutionizing Language Processing: Quen's Flexible Text Embeddings
Quen introduces cutting-edge text embeddings on HuggingFace, offering flexibility and customization. Ranging from 6B to 8B in size, these models excel in benchmarks and support instruction-based embeddings and reranking. Accessible for local or cloud use, Quen's models pave the way for efficient and dynamic language processing.
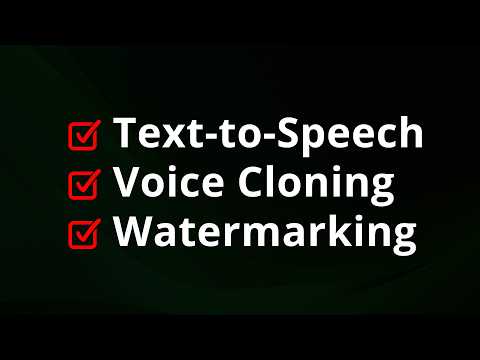
Unleashing Chatterbox TTS: Voice Cloning & Emotion Control Revolution
Discover Resemble AI's Chatterbox TTS model, revolutionizing voice cloning and emotion control with 500M parameters. Easily clone voices, adjust emotion levels, and verify authenticity with watermarks. A versatile and user-friendly tool for personalized audio content creation.