Revolutionizing AI Reasoning Models: The Power of a Thousand Examples
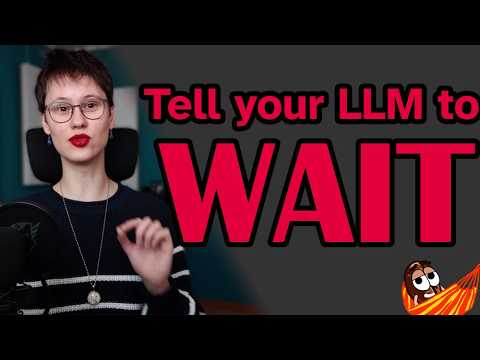
- Authors
- Published on
- Published on
In this thrilling episode of AI Coffee Break with Letitia, we delve into a groundbreaking paper that shakes the very foundations of AI reasoning models. Forget about needing a gazillion examples to train these beasts like DeepSeek R1; turns out, all you need is a carefully curated thousand examples. But wait, there's more! The ingenious use of a test time compute trick ensures these models are firing on all cylinders when it comes to churning out those crucial reasoning chains.
The team behind this revolutionary approach takes us on a wild ride through the world of distillation, fine-tuning their model, S1, on a selection of mind-bending questions from various Olympiads and standardized tests. By weeding out the weak and favoring the tough nuts to crack, they create a dataset that pushes their model to the limits. And boy, does it deliver! S1 flexes its 32 billion parameters muscles and outshines the competition, leaving giants like OpenAI in its dust.
But hold on to your seats, folks, because the excitement doesn't stop there. Test time scaling swoops in to save the day, with a clever little trick involving the word "wait" that turbocharges S1's reasoning accuracy. This budget forcing method is like giving your AI a shot of adrenaline, pushing it to double-check and refine its answers like a pro. However, as with all good things, there's a catch – longer reasoning chains mean higher computational costs. It's a high-stakes game of accuracy versus speed, and the question remains: how far are we willing to push the limits for smarter, faster answers?
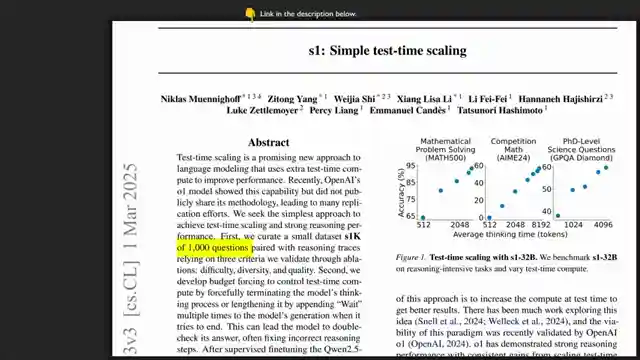
Image copyright Youtube
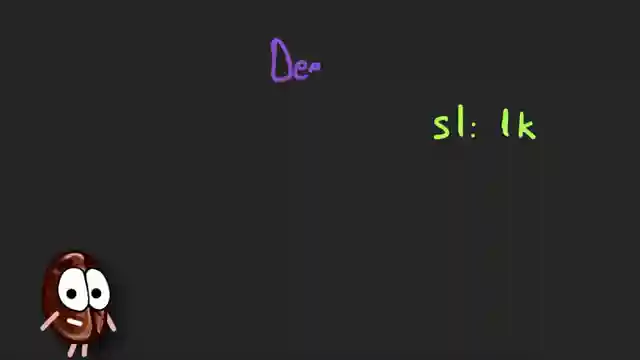
Image copyright Youtube
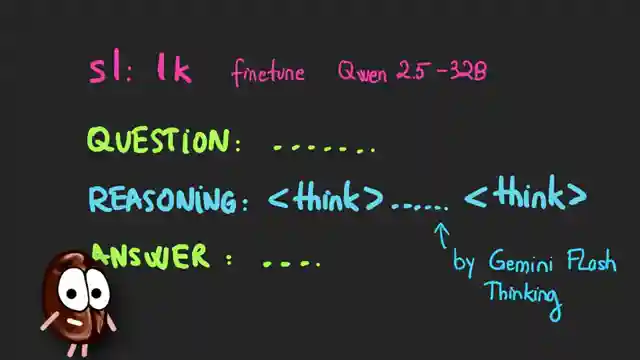
Image copyright Youtube
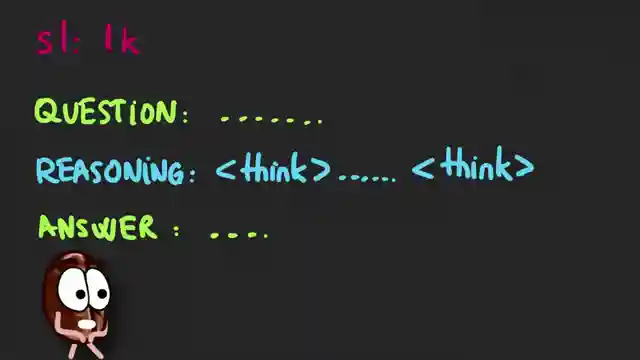
Image copyright Youtube
Watch s1: Simple test-time scaling: Just “wait…” + 1,000 training examples? | PAPER EXPLAINED on Youtube
Viewer Reactions for s1: Simple test-time scaling: Just “wait…” + 1,000 training examples? | PAPER EXPLAINED
Viewers are impressed by the clever tricks used to enhance reasoning and increase performance
Fei Fei Li's involvement is noted and the importance of quality samples is mentioned
Excitement over a new AI Coffee Break video
Appreciation for the video and its method of fine-tuning training data to help the LLM track human logic in questions
Excitement over the return of a specific individual
Impatience for the next video
Mention of Diffusion LLMs requiring more steps for correct response
Request for a video on test-time compute
Suggestions for adding phrases to direct the model to consider all possible ways of thinking
Discussion on research being done on test time compute efficiency and the importance of using fewer words in each step
Comment on distilling Gemini's reasoning traces and the importance of high-quality seed reasoning traces in the paper's presentation.
Related Articles
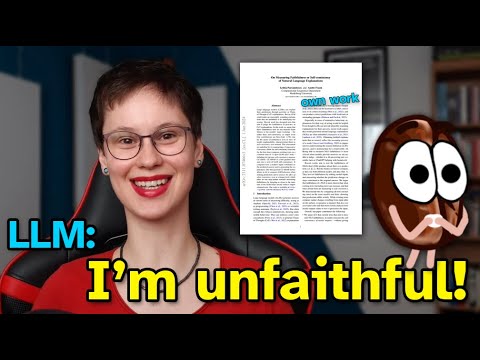
Revolutionizing Model Interpretability: Introducing CC-SHAP for LLM Self-Consistency
Discover the innovative CC-SHAP score introduced by AI Coffee Break with Letitia for evaluating self-consistency in natural language explanations by LLMs. This continuous measure offers a deeper insight into model behavior, revolutionizing interpretability testing in the field.
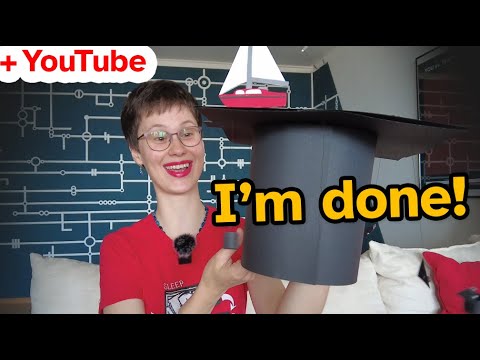
PhD Journey in Image-Related AI: From Heidelberg to Triumph
Join AI Coffee Break as the host shares her captivating PhD journey in image-related AI and ML, from Heidelberg to deep learning research, collaborations, teaching, and the triumphant PhD defense. A tale of perseverance, growth, and academic triumph.
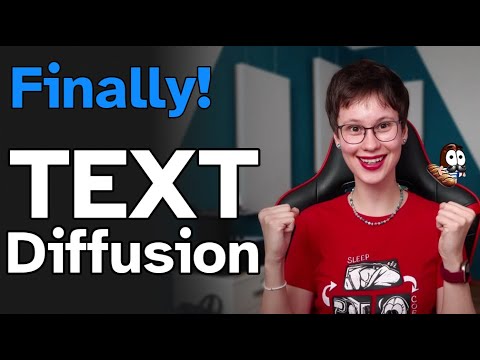
Revolutionizing Text Generation: Discrete Diffusion Models Unleashed
Discover how discrete diffusion models revolutionize text generation, challenging autoregressive models like GPT with improved coherence and efficiency. Explore the intricate process and promising results of SEDD in this AI Coffee Break episode.
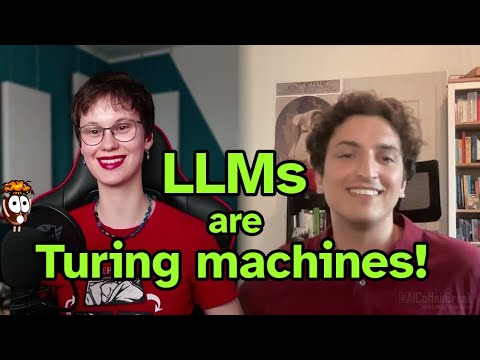
Unveiling the Power of Transformer Architectures in Language Modeling
Discover how Transformer architectures mimic Turing machines and how Transformers with Chain of Thought can simulate probabilistic touring machines, revolutionizing language models. France Novak explains the computational power of llm architectures in natural language processing.