Enhancing Calorie Estimation Accuracy with Die GPT: Human Supervision and Food Databases
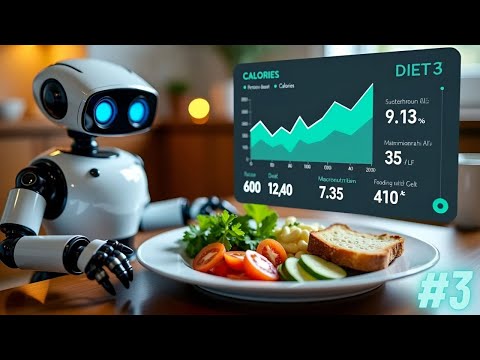
- Authors
- Published on
- Published on
In this riveting installment from Aladdin Persson, the die GPT series delves into the nitty-gritty of calorie estimation accuracy. The team embarks on a mission to scrutinize the model's performance by crafting insightful graphs using CSV data from their previous escapade. Armed with metrics like mean errors and percentage errors, they aim to unravel the model's prowess in a visually compelling manner. As they dissect extreme cases of overestimates and underestimates, the team uncovers the model's Achilles' heel, paving the way for crucial insights.
With a flair for the dramatic, Aladdin Persson's crew unveils a tale of triumphs and tribulations in the realm of calorie estimation. Despite the model's tendency to overestimate by a mere eight calories on average, the devil lurks in the details with staggering errors of up to 300 calories. Through the lens of key metrics like R squared and mean error, the team navigates the treacherous waters of model accuracy, hinting at a promising yet volatile landscape. The call for human intervention rings loud and clear as they advocate for a symbiotic relationship between man and machine to achieve the pinnacle of precision.
As the dust settles on this adrenaline-fueled episode, Aladdin Persson's band of tech enthusiasts teases a tantalizing future. Plans are afoot to fuse human oversight with the model's computational wizardry, promising a harmonious blend of intuition and accuracy. The stage is set for a grand experiment, where the fusion of human intellect and artificial intelligence may unlock a new realm of possibilities. In the upcoming saga, the team vows to elevate the model's capabilities by integrating a comprehensive food database, heralding a new era of nuanced macronutrient analysis and calibrated calorie predictions.
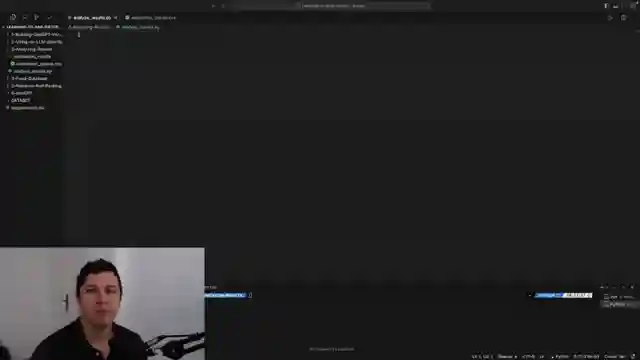
Image copyright Youtube
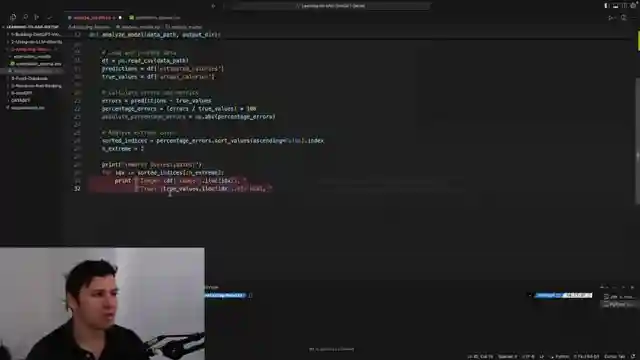
Image copyright Youtube
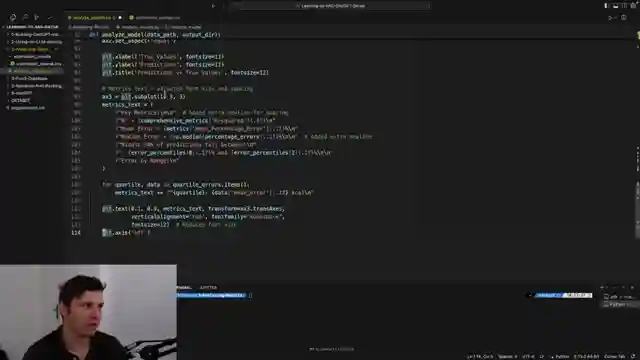
Image copyright Youtube
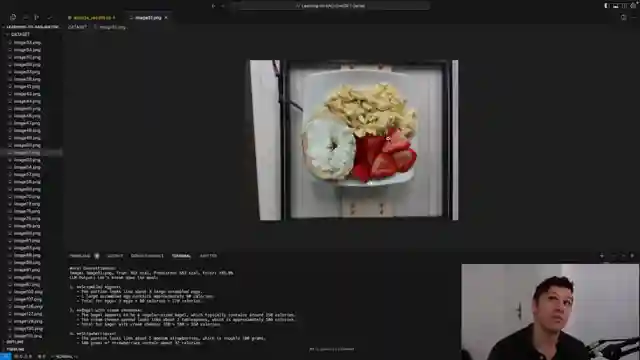
Image copyright Youtube
Watch Building DietGPT - Evaluating Model (Learning to build RAG series) #3 on Youtube
Viewer Reactions for Building DietGPT - Evaluating Model (Learning to build RAG series) #3
Inquiry about the source of the test data set
Praise for the work done
Question about how the code appeared line by line
Related Articles
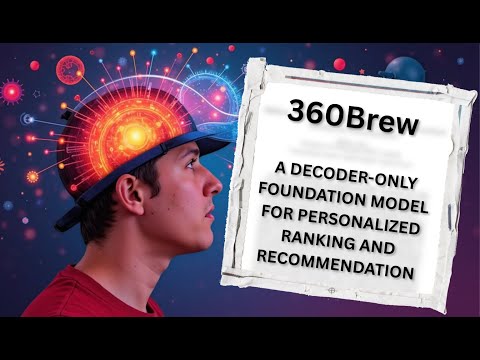
Revolutionizing Recommendations: 360 Brew's Game-Changing Decoder Model
Aladdin Persson explores a game-changing 150 billion parameter decoder-only model by the 360 Brew team at LinkedIn, revolutionizing personalized ranking and recommendation systems with superior performance and scalability.
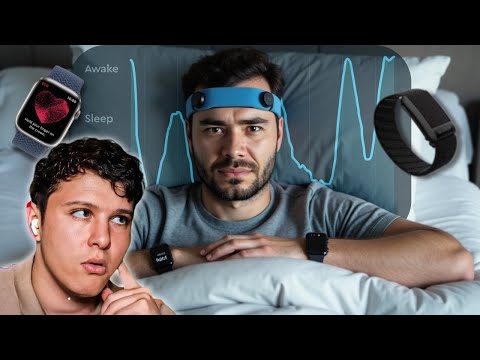
Best Sleep Tracker: Whoop vs. Apple Watch - Data-Driven Insights
Discover the best sleep tracker as Andre Karpathy tests four devices over two months. Whoop reigns supreme, with Apple Watch ranking the lowest. Learn the importance of objective data in sleep tracking for optimal results.

Mastering Self-Supervised Learning: Fine-Tuning DNOV2 on Unlabeled Meme Data
Explore self-supervised learning with DNOV2 and unlabeled meme data in collaboration with Lightly Train. Fine-tune models effortlessly, generate embeddings, and compare results. Witness the power of self-supervised learning in meme template discovery and potential for innovative projects.
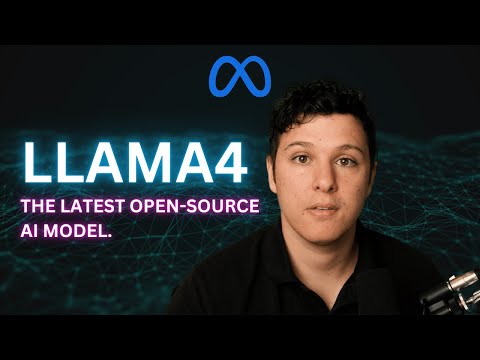
Unveiling Llama 4: AI Innovation and Performance Comparison
Explore the cutting-edge Llama 4 models in Aladdin Persson's latest video. Behemoth, Maverick, and Scout offer groundbreaking AI innovation with unique features and performance comparisons, setting new standards in the industry.