Demystifying Data Science Roles: MLEs, Analysts, and Python Skills
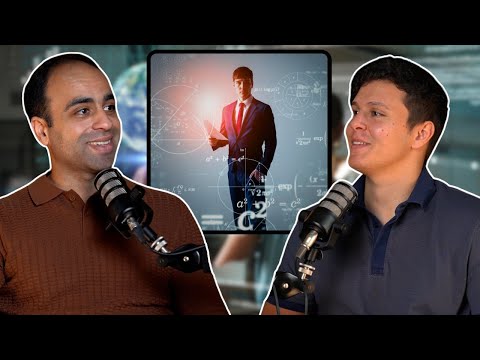
- Authors
- Published on
- Published on
In this riveting discussion, Aladdin Persson delves into the dynamic world of data science roles, from the meticulous analysts to the ingenious AI engineers. The spotlight shines on the Machine Learning Engineers (MLEs), the unsung heroes who master the art of efficient code implementation. Meanwhile, data scientists take center stage with their prowess in modeling and deep learning, a domain where Google often seeks statisticians to crunch the numbers. The interview process is no walk in the park, with a heavy emphasis on statistics that can make or break one's shot at landing a data science gig.
Data analysts, on the other hand, navigate the realm of analytics, visualization, and basic modeling, their SQL skills serving as a compass in the data landscape. The distinction between data scientist and analyst roles can be as blurry as a foggy British morning, making it crucial to scrutinize job descriptions before diving in. Python proficiency is the golden ticket for data scientists, although familiarity with other languages might not be a deal-breaker. The discussion also unveils the dichotomy between product-focused and research-focused data science roles, each demanding a unique set of skills and presenting distinct career trajectories.
Transitioning between different data science realms is akin to maneuvering a treacherous mountain pass, requiring careful consideration of one's career aspirations and the responsibilities tethered to each role. Aladdin Persson's insights shed light on the intricate web of data science roles, urging aspiring data enthusiasts to chart their course wisely in this ever-evolving landscape.
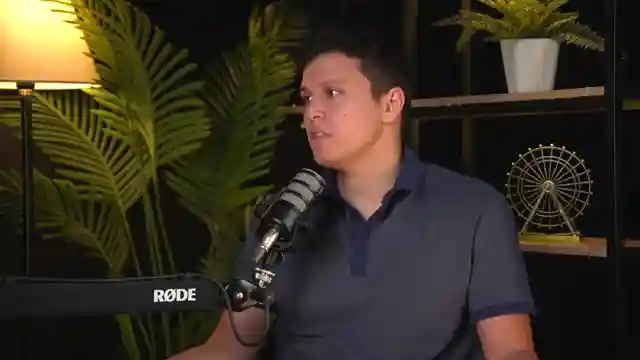
Image copyright Youtube
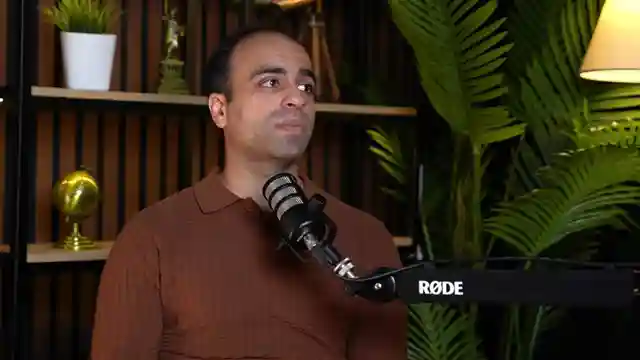
Image copyright Youtube
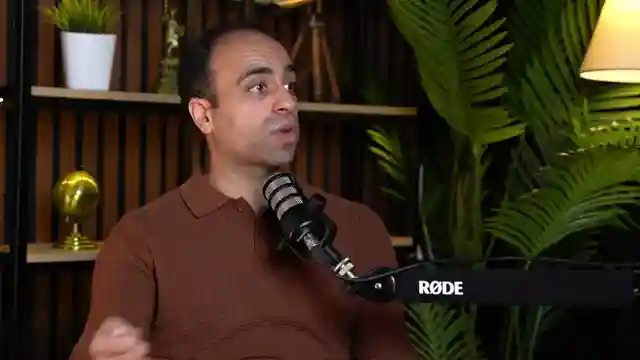
Image copyright Youtube
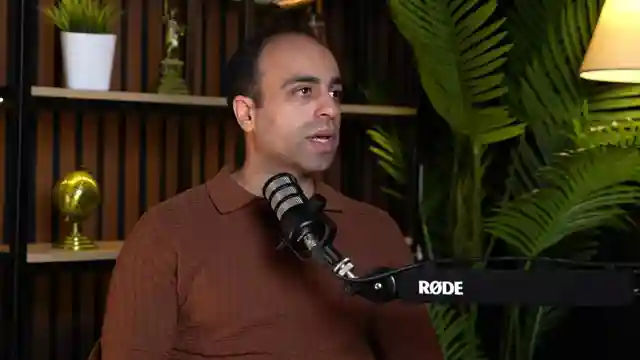
Image copyright Youtube
Watch What’s the difference between Data Analyst, MLE and Data Scientist role on Youtube
Viewer Reactions for What’s the difference between Data Analyst, MLE and Data Scientist role
Request for a tutorial on installing Isaac Sim for RL applications on Windows
Comment on overlapping categories in the field, such as transitioning from machine learning models to applying LLMs in business applications.
Related Articles
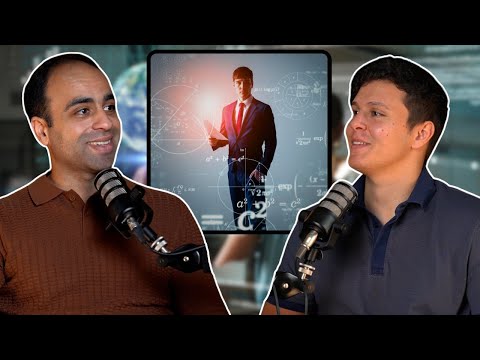
Demystifying Data Science Roles: MLEs, Analysts, and Python Skills
Explore the diverse roles in data science, from MLEs to data scientists, and the importance of skills like Python and SQL. Understand the differences between analytics and modeling-focused positions for a successful career in this dynamic field.
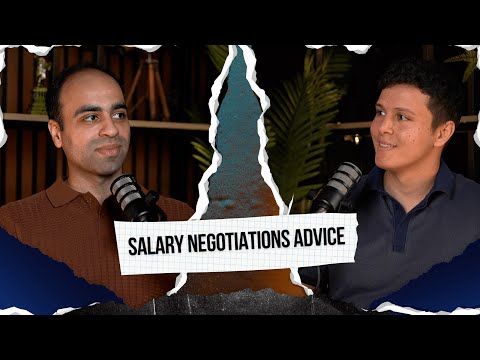
Mastering Salary Negotiations: Strategies for Securing Your Worth
Learn the art of salary negotiations from Aladdin Persson's insightful video. Discover how to avoid lowball offers, strategically counter, and secure a deal that reflects your true value in the job market. Master the negotiation game and maximize your earning potential.
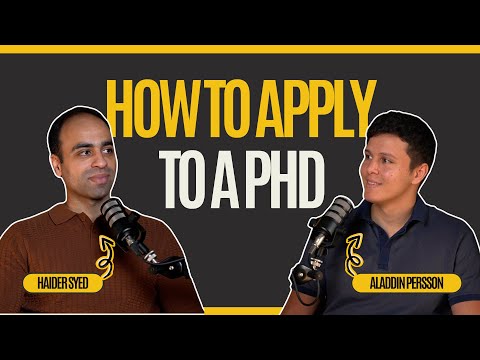
Maximizing PhD Acceptance: Contacting Professors for Success
Aladdin Persson provides expert advice on maximizing PhD program acceptance chances by contacting professors directly, showcasing research experience, and demonstrating passion and commitment during interviews.
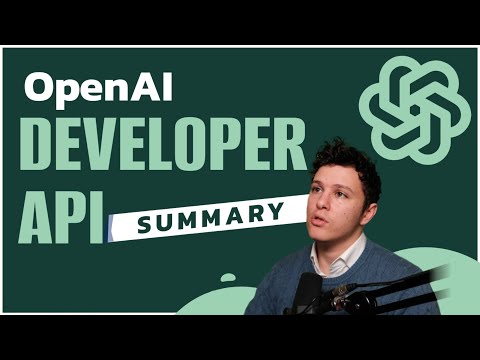
Mastering OpenAI GPT-4: Practical Tips for Efficient API Usage
Aladdin Persson shares practical tips for utilizing the OpenAI GPT-4 API efficiently, from setting up the API to navigating rate limits and optimizing multi-threading for faster processing. Dive into the world of AI innovation with expert guidance.